Mariusz Slowik
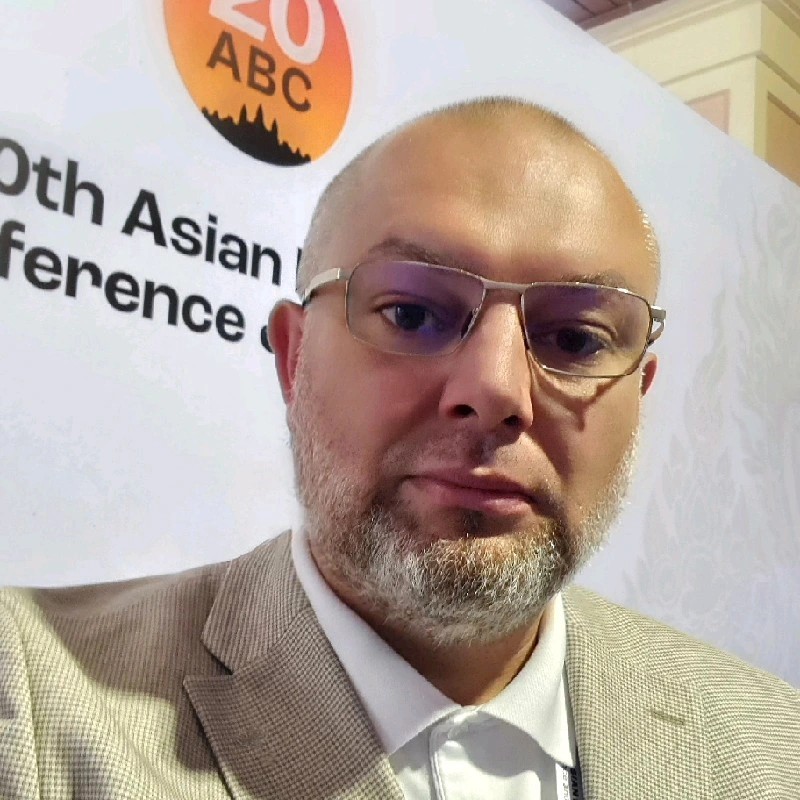
Bio
Mariusz Słowik is a graduate of Wrocław University of Science and Technology, holding a degree in Mechanical-Energy Engineering. He furthered his education with an Executive MBA from the University of Economics of Wrocław. Additionally, Mariusz studied Process Management at the Leon Koźmiński Academy, where he pursued a PhD program in Management, with a wealth of professional experience in operational process management within large industrial factories. Mariusz has spent the last 14 years at AUTOPART as the Vice President of the Board. In this role, he is responsible for overseeing production processes, procurement, logistics, maintenance, and continuous improvement.
In the dynamic landscape of energy transformation, ensuring a significant role for lead batteries necessitates continuous refinement not only in design, raw materials, but also in production methods. For over 30 years, AutoPart has been manufacturing batteries utilizing lead oxide from Barton reactors, employing some of the world’s largest installations of this kind. Despite decades of experience, lead oxide production remains a challenge in this technology due to its high inertia and the multitude of factors influencing the process. Achieving desired oxide parameters requires extensive operator knowledge and intuition. While literature provides insights into lead oxide requirements, operational parameters for Barton reactors defining oxide properties are lacking. Leveraging its expertise, AutoPart has initiated research endeavours through two implementation-focused doctoral projects aimed at developing technological parameters for Barton reactor operation to produce lead oxide with programmable properties. Additionally, the integration of machine learning algorithms in process control is explored, aiming to limit operator intervention to supervisory tasks. This envisioned change aims to enable predictive process management through artificial intelligence, thereby minimizing deviations in crucial lead oxide parameters. This material presents research findings on factors influencing lead oxide production processes and outcomes related to the application of artificial intelligence in process control.